Table of Contents
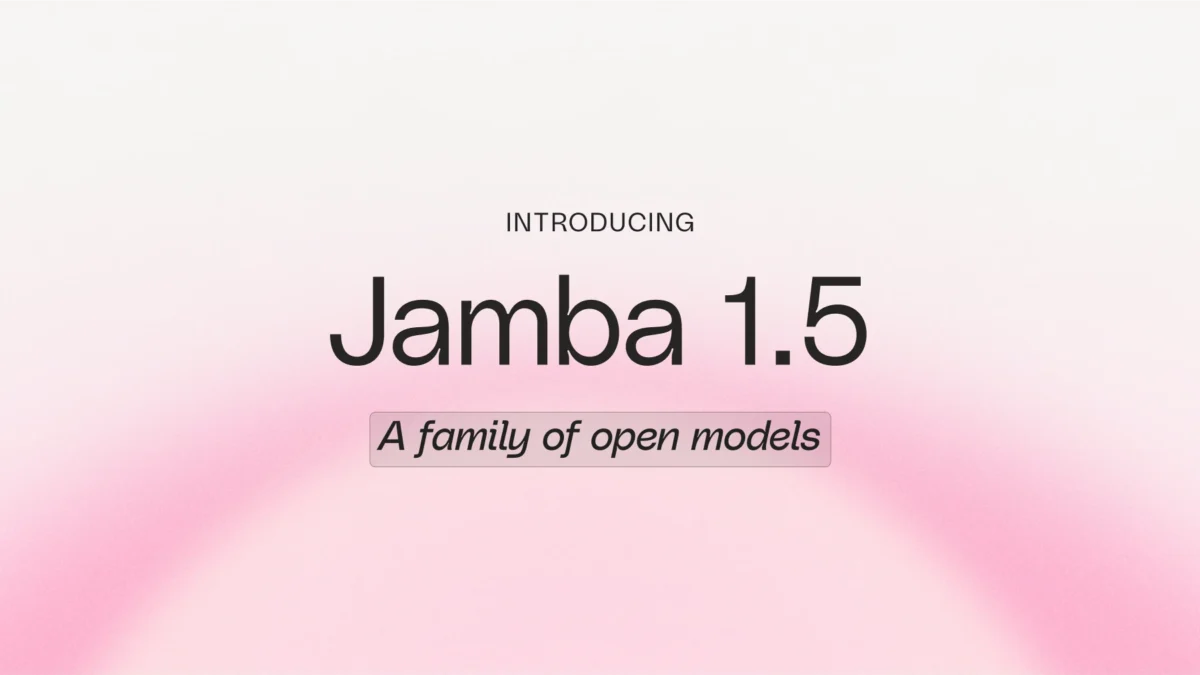
The Jamba 1.5 Open Model Family: The Most Powerful and Efficient Long Context Models
Today, we are debuting the Jamba 1.5 family of open models: Jamba 1.5 Mini and Jamba 1.5 Large. Built on our novel SSM-Transformer architecture, these models demonstrate superior long context handling, speed, and quality—outranking competitors in their size class and marking the first time a non-Transformer model has been successfully scaled to the quality and strength of the market’s leading models.
We are releasing these models under the Jamba Open Model License, upholding our commitment to democratizing access to quality models and opening the door to further experimentation. Find a detailed description of the model’s architecture and all evaluation results in the whitepaper.
Today’s language models are impressive in their capabilities—but too often fail to deliver real value for businesses.
At AI21, we are on a mission to change this by designing AI systems that are purpose-built for the enterprise. These models are built keeping in mind the key measures large businesses care most about when it comes to GenAI implementation: resource efficiency, quality, speed, and ability to actually solve critical tasks.
- Long context handling: With a 256K effective context window, the longest in the market, Jamba 1.5 models can improve the quality of key enterprise applications, such as lengthy document summarization and analysis, as well as agentic and RAG workflows
- Speed: Up to 2.5X faster on long contexts and fastest across all context lengths in their size class
- Quality: Jamba 1.5 Mini is the strongest open model in its size class with a score of 46.1 on the Arena Hard benchmark, surpassing larger models like Mixtral 8x22B and Command-R+. Jamba 1.5 Large, with a score of 65.4, outpaces both Llama 3.1 70B and 405B
- Multilingual: In addition to English, the models support Spanish, French, Portuguese, Italian, Dutch, German, Arabic and Hebrew
- Developer ready: Jamba natively supports structured JSON output, function calling, digesting document objects, and generating citations
- Open for builders: Both models are available for immediate download on Hugging Face (and coming soon to leading frameworks LangChain and LlamaIndex)
- Deploy anywhere: In addition to AI21 Studio, the models are available on cloud partners Google Cloud Vertex AI, Microsoft Azure, and NVIDIA NIM and coming soon to Amazon Bedrock, Databricks Marketplace, Snowflake Cortex, Together.AI as well as for private on-prem and VPC deployment
Resource-efficient hybrid architecture
Jamba 1.5 Large and Mini are built on the novel SSM-Transformer Jamba architecture, which weaves together Transformer’s outstanding quality with Mamba’s groundbreaking efficiency.
As a result, the models offer a lower memory footprint than competitors, allowing clients to handle context lengths up to 140K tokens on a single GPU using Jamba 1.5 Mini. The same advantage also makes fine-tuning over long contexts easier and more accessible than with transformer-based models. Thanks to this efficiency-optimized architecture, our models can deliver top quality and speed without skyrocketing costs.
Like all models in its size class, Jamba 1.5 Large can’t be loaded in full (FP32) or half (FP16/BF16) precision on a single node of 8 GPUs. Dissatisfied with currently available quantization techniques, we developed ExpertsInt8, a novel quantization technique tailored for MoE models.
With ExpertsInt8, we only quantize weights that are parts of the MoE (or MLP) layers, which for many MoE models account for over 85% of the model weights. In our implementation, we quantize and save these weights in INT8, an 8-bit precision format, and dequantize them at runtime directly inside the MoE GPU kernel.
This technique offers four advantages: It is fast, with quantization taking up to just a few minutes; it does not rely on calibration, a sometimes unstable process which ordinarily can take hours or days; it can still use BF16 to hold large activations; and, importantly, it allows Jamba 1.5 Large to fit on a single 8 GPU node, while utilizing its full context length of 256K. In our experiments, ExpertsInt8 proved to have the lowest latency of all vLLM quantization techniques for MoE models, without a loss in quality.
Long context that actually delivers
The 256K context window offered by the Jamba 1.5 models is not only the longest amongst open models, but also the only one to back this claim on the RULER benchmark.
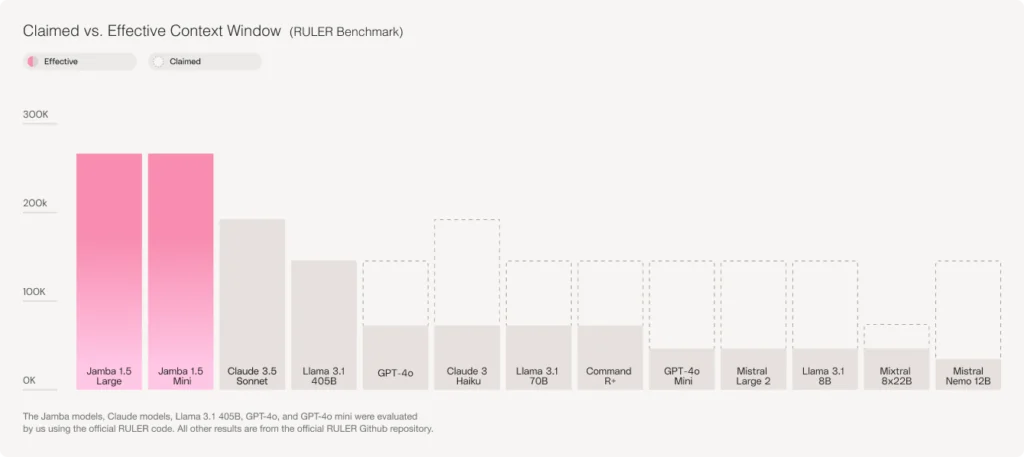
Whereas most other models claim a long context window but fail to sustain the same quality of performance at the upper limits of their context window, the Jamba 1.5 family maintains its long context handling throughout the entire span of its 256K context window.
A model that can effectively handle long context is crucial for almost every enterprise scale GenAI application. In addition to thoroughly and precisely summarizing and analyzing lengthy documents, a long context model substantially improves the quality of RAG and agentic workflows—and reduces their cost—by eliminating the need for continuous chunking and repetitive retrievals.
While it’s sometimes claimed that RAG is a substitute for long context, a successful enterprise AI system needs both. In pairing long context and RAG, the long context model improves the quality and cost-efficiency of RAG’s retrieval stage at scale.
Fastest on the market
For the use cases enterprises are interested in, such as customer support agent assistants and chatbots, rapid turnaround is essential. The model needs to be able to keep pace with the scale of operations, even as usage requests and batch sizes increase.
Both Jamba 1.5 models are faster than competitors of a similar size, with up to 2.5X faster inference on long contexts, offering customers major cost, quality, and speed gains under high utilization when deployed in their own environment.
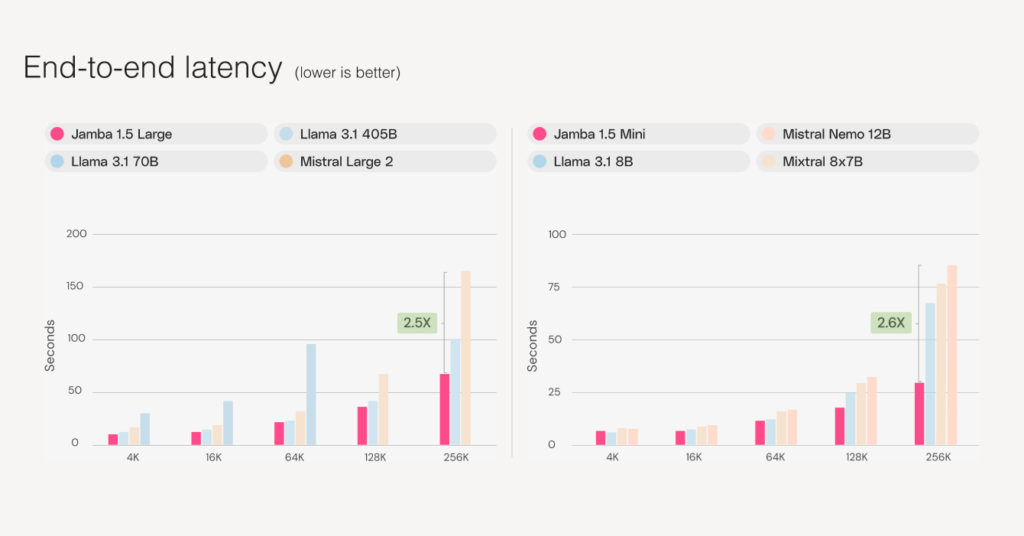
Jamba 1.5 Mini and Jamba 1.5 Large show excellent speed and throughput results in tests run by Artificial Analysis, as can be seen in the chart below, with Jamba 1.5 Mini ranking as the fastest model on 10K contexts.
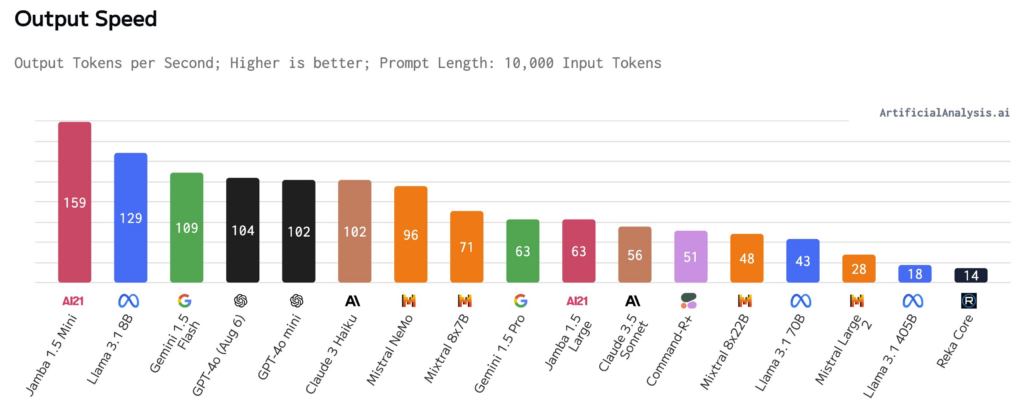
Outstanding quality across the board
As measured on the Arena Hard benchmark, Jamba 1.5 Mini emerges as the strongest model in its size class, outshining competitors Claude 3 Haiku, Mixtral 8x22B and Command-R+. Jamba 1.5 Large similarly rises above leading models like Claude 3 Opus, Llama 3.1 70B, and Llama 3.1 405B, offering excellent value per cost for its size class. Find all evaluations in Section 6 of the whitepaper.
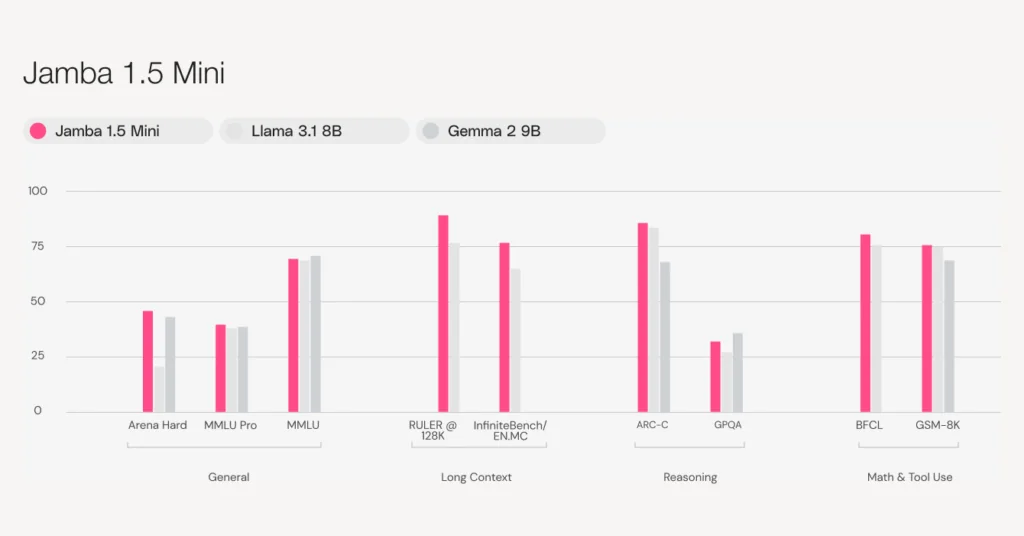
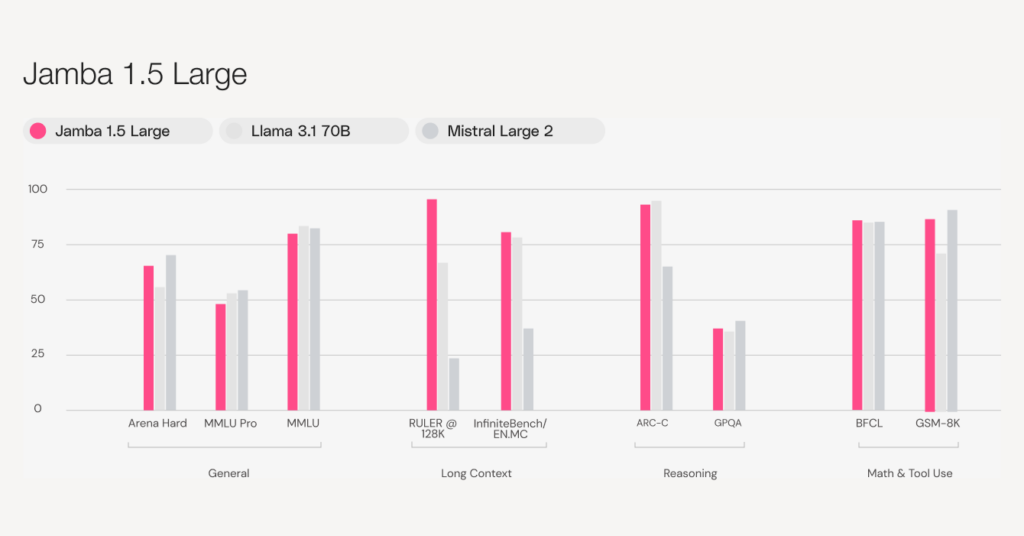
Getting started
Build with Jamba 1.5 Mini or Jamba 1.5 Large wherever you like to work. The models are available on the following platforms and cloud partners:
And coming soon to Amazon Bedrock, Databricks Marketplace, LangChain, LlamaIndex, Snowflake Cortex, and Together.AI.
For customers who wish to avoid a lengthy experimentation process and keep their data onsite, we offer private deployments and custom models. In this white-glove service, we tailor our models exactly to your needs and use case through continuous pre-training and fine-tuning so you can move more quickly from ideation to production.
To learn more about how Jamba 1.5 Mini and Jamba 1.5 Large can bring real world value to your organization, let’s talk.