Table of Contents
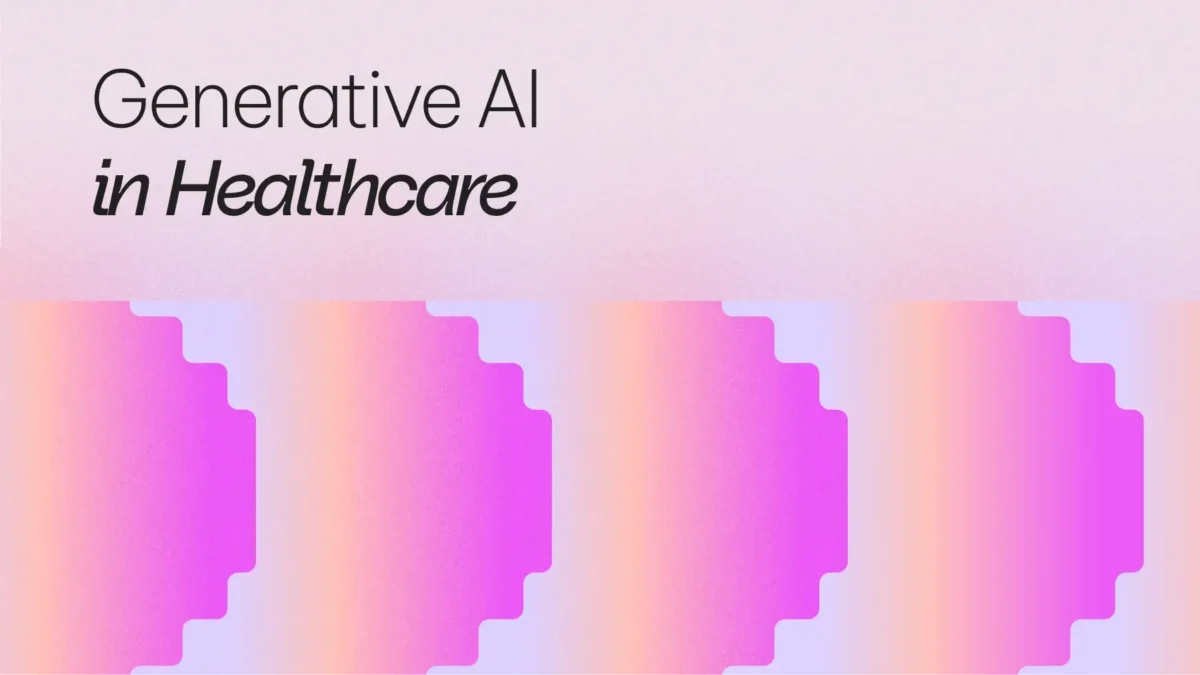
Generative AI in Healthcare: Applications, Examples & Benefits
Burnout, backlogs, and budget pressure continue to stretch care teams thin, particularly in underserved communities.
Clinicians spend nearly half their day on documentation rather than with patients, and these inefficiencies are more than operational headaches; they hinder patient care.
Generative AI offers a new path forward by automating clinical documentation, surfacing insights from unstructured data, and enhancing medical imaging for more efficient, personalized, and equitable care.
But adoption must be intentional, compliant, and trustworthy. This article explores what generative AI really means for healthcare, how it works, and what enterprise providers need to know.
What is generative AI in healthcare?
Generative AI refers to a class of machine learning models capable of producing original content, text, images, video, or structured data based on learning patterns from vast training data or enterprise-specific datasets.
In healthcare, generative AI can be used to automate and enhance clinical tasks using unstructured data sources, for example:
- Summarizing patient notes.
- Transcribing voice recordings.
- Interpreting images in medical reports and scans.
- Generating plain-language instructions for patients.
It excels at making sense of the data that doesn’t fit neatly into spreadsheets or drop-down menus, such as doctors’ notes, radiology reports, pathology slides, or audio from patient consultations. It can extract meaning from this messy, nuanced data without requiring teams to sift through files and transcripts manually.
Plus, techniques like Retrieval-Augmented Generation (RAG), which improve language models by pulling in real-time information from external sources, make these tools even more reliable by ensuring generative AI is grounded in trusted sources, like peer-reviewed research or institutional guidelines, so outputs are more likely to be relevant and accurate.
Why is generative AI in healthcare gaining attention?
A 2025 study of 1,500 physicians found that more than half of the physicians surveyed believed AI tools could be ‘somewhat or very helpful’ in healthcare areas.
The most popular proposed applications were in diagnostic ability (72%), clinical outcomes (62%), care coordination (59%), patient convenience (57%), patient safety (56%), resource allocation of staff (56%), and revenue (54%).
Specific areas identified, all of which are possible at scale using generative AI, include:
- Enhancing billing codes, visit notes, and medical charts.
- Creating discharge instructions, care plans, or progress notes.
- Drafting responses to patient portal messages.
- Automating prior authorization for insurance.
- Translating patient communications.
- Summarizing medical research and care guidelines.
With many of these tasks being repetitive and labor-intensive, generative AI offers a way for healthcare professionals to have more time for the tasks that matter.
Generative AI applications for healthcare
Generative AI is already transforming healthcare in areas as vast as patient engagement to research. Here are just a few of the real-world applications used in healthcare settings.
Clinical note analysis
Studies suggest that up to 80% of medical data is unstructured. The fact is that data that can’t be identified, let alone measured, has an increased risk of being overlooked. This can result in missing intelligence from patient histories or failing to spot potential drug interactions.
Generative AI extracts key medical terms, medications, lifestyle guidance, and even social determinants of health from medical documents and systems, or analysis of voice or handwritten files, capturing details that are often absent or overlooked in electronic health records (EHRs).
Tools like AI21 Labs’ Contextual Answers, which use RAG, can even enable clinicians to ask natural-language questions about any clinical documents and receive grounded, document-based responses, for example, ‘Will this patient react negatively to this medicine given their history?’ This RAG-focused approach not only reduces hallucinations and builds trust with healthcare users but also improves review times by up to 40%.
Generate clinical trial materials
Generative AI supports the creation of clinical trial materials, particularly in evaluation brochures used in pharmaceutical trials.
These must be adapted for different audiences, such as clinicians, regulators, and patients, each requiring tailored messaging and appropriate language.
This previously time-consuming task is being done with Generative AI tools, which help summarize previous studies, incorporate the latest trial results automatically, and ensure information is clear and jargon-free for patient-facing materials, improving comprehension while still meeting compliance and regulatory standards.
Removing barriers to healthcare
Nearly half of U.S. adults said that they had difficulty affording healthcare costs, and while four in ten U.S. adults have some type of health care debt, disproportionate amounts of lower-income adults, the uninsured, Black and Hispanic adults, women, and parents report their current debt is due to medical costs.
Generative AI is removing some of these obstacles. For example, telemedicine and remote monitoring analyze patient data and flag potential health concerns in real time, with models such as Jamba supporting multilingual outputs (e.g., Arabic, Hebrew, Spanish).
Using Conversational AI, a type of artificial intelligence that simulates human-like discussions using natural language processing (NLP) and machine learning techniques, patients can chat with doctors, instantly enabling wider patient access to trusted health information, overcoming language-based inequities.
The efficiencies generative AI offers also mean admin is reduced, and time is better allocated. By automatically assigning medical codes for billing, streamlining prior authorization requests, finding patient records, and identifying risks, insurance payouts can be arranged sooner.
Image analysis
One of the most promising applications of generative AI in healthcare is image enhancement and reconstruction, offering the ability to spot the warning signs of significant diseases such as cancers.
Generative AI excels at pattern recognition, making it ideal for extracting biomarkers and measurements from images, as well as critical inputs for timely, accurate diagnoses. Healthineers’ AI-Rad Companion Chest CT software provides automated measurements of lung nodules in patients, but AI models can be used to improve the clarity of medical images and detect subtle changes from existing CT and MRI scans. This reduces the risk of human error in identifying subtle changes everywhere, from an oncology ward to the ultrasounds used in fetal monitoring.
Relieving the admin burden of electronic health care records
Electronic Health Records (EHRs) are frequently cited as a key contributor to clinician burnout. Much of the data stored in EHRs exists as unstructured text within clinical notes, making it time-consuming to extract insights.
Generative AI, powered by Natural Language Understanding (NLU) – machine learning that’s able to understand and interpret human language, and conversational AI can help surface important details, eliminating the need to sift through lengthy records manually.
For faster follow-ups after patient visits, generative AI also automates note transcription, applies medical ontologies to label key terms, and transforms documents into structured tables. It can also analyze EHR content to suggest accurate medical codes, supporting smoother pre-authorization processes for insurance purposes, monitoring for approvals, denials, or requests for additional information.
Real-world examples of generative AI in healthcare
Generative AI generates complex outputs from input data, but that doesn’t just mean text or images! It’s being used to work with proteins to speed up drug discovery, and it’s changing research methods, reducing time and costs.
AWS Batch with Novo Nordisk is using generative AI to enhance protein structure prediction, asking the generative AI to ‘learn’ the underlying biological rules needed to generate accurate structural predictions for unknown proteins.
Prior to using generative AI, this research was only possible with time-consuming and expensive methods like X-ray crystallography.
Generative AI is also being adopted in pharmaceuticals, helping improve quality control in drug manufacturing and speeding up product release. With industry typical false drug rejection rates between 1 and 45%, costing the industry up to $740 million annually, generative AI offers a tremendous opportunity.
It can generate synthetic images of defects to train data and help AI models detect only real defects and reduce false rejections, as shown in the case of Merck and AWS.
Challenges and limitations of generative AI in healthcare settings
Generative AI offers the promise of freeing healthcare professionals from repetitive administrative tasks and allowing more time for direct patient care to those who need it or have been overlooked. However, as adoption grows, so too do the challenges.
Healthcare organizations must carefully consider the legal, ethical, and operational risks of deploying generative AI systems in clinical environments. Here are a few to consider.
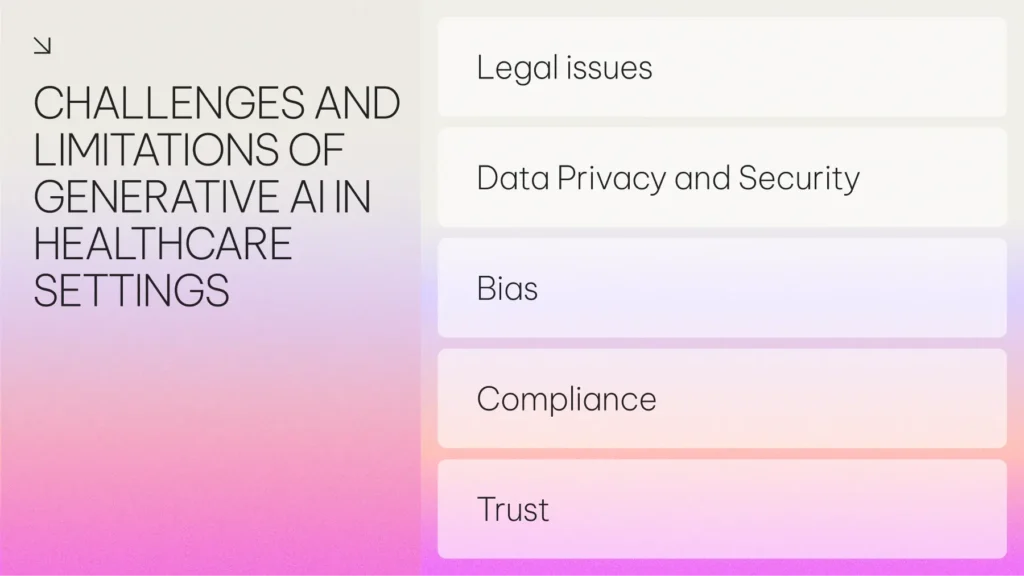
Legal issues
A core concern is legal responsibility. When any AI systems make incorrect recommendations or generate flawed outputs that contribute to adverse patient outcomes, it’s vague as to who is liable. While the final decision typically rests with the clinician, this gray area raises questions about accountability.
Data Privacy and Security
Generative AI systems process large volumes of sensitive data, making compliance with privacy laws like HIPAA essential. Any use of AI tools, especially those hosted by third parties, must not violate patient confidentiality.
One solution is using synthetic data, such as medical images generated by Generative Adversarial Networks (GANs). This is a type of AI where two models compete to produce highly realistic outputs, which can help protect patient privacy because the images are artificial and not linked to actual individuals.
Bias
Generative AI models inherit and amplify biases present in their training data, leading to skewed or discriminatory outputs. These issues can perpetuate healthcare inequities, particularly among underrepresented populations.
Organizations like the Coalition for Health AI and FaaCT recommend rigorous fairness evaluations to ensure algorithms are tested across diverse demographics before clinical use.
Compliance
Patient trust depends on transparency. Individuals should be informed when their data is used in AI systems and must give explicit consent, especially when data may be shared externally or across borders via cloud infrastructure. Failing to do so not only undermines trust but also risks regulatory breaches.
Trust
It’s well known that generative AI outputs, especially from broad, large language models, can “hallucinate” facts, producing outputs that appear credible but are incorrect.
While efforts like RAG help improve traceability and reduce hallucinations, all generative AI systems must be designed with human oversight as core to their use.
The future for healthcare, powered by generative AI
Generative AI is no longer experimental. It’s already delivering measurable results in healthcare — so the challenge now isn’t whether to adopt AI, but how to do so responsibly.
The most secure and scalable approach to deploying generative AI in healthcare is through private AI deployment and containerized model management. This often involves running models in a virtual private cloud (VPC) using platforms like Amazon Elastic Kubernetes Service (EKS).
This setup gives healthcare organizations full control over where data is stored, how models are managed, and who has access — all critical for meeting internal compliance, legal, and data governance standards.
It mirrors the infrastructure many regulated industries already use to handle sensitive data, and it enables healthcare providers to process clinical information securely — ensuring that models can be fine-tuned or updated without relying on third-party cloud providers.
AI is not designed to replace clinical judgment, but to enhance it — helping healthcare professionals move faster, detect patterns earlier, and make confident decisions without missing what matters, especially when serving the communities most in need.