Table of Contents

Generative AI in Finance: Applications, Examples & Benefits
Finance leaders are under growing pressure to deliver insights faster, improve agility, and make smarter, data-driven decisions. Yet many are still held back by a cautious approach to AI adoption, leaving them vulnerable as competitors accelerate ahead.
KPMG has found 83% of finance teams are using AI to support predictive modeling and scenario analysis, and a recent McKinsey report estimates that gen AI could add between $200 billion and $340 billion in value across the global banking sector.
To unlock this value securely, finance leaders can use generative AI, with private, fine-tuned AI deployments that reduce unnecessary risk.
This article breaks down the most valuable use cases of this approach, as well as guidance on how to implement generative AI responsibly.
What is generative AI for finance?
Generative AI is a class of artificial intelligence models that generate original content like text, images, and even code based on patterns in data.
The use cases for finance professionals are vast, ranging from summarizing complex reports, identifying trends, detecting anomalies, and generating insight-rich commentary at speed, but that’s just the start. Generative AI can handle unstructured data, making it ideal for support tasks such as:
- AI-powered chatbots for customer service.
- Anomaly detection for fraud prevention.
- Predictive models for financial reporting.
- Tailored investment strategy generation.
- Intelligent document summarization.
- Workflow automation across FP&A, trading, and insurance.
Advanced models with a longer context window possess even greater capabilities to process lengthy, complex financial documents, which are critical for applications such as 10-K analysis, investor decks, and scenario modeling.
Additionally, with the aid of Retrieval-Augmented Generation (RAG), a technique that enhances generative AI models by connecting them to trusted external or internal data, these models can generate responses grounded in policy, regulation, and historical data, resulting in more accurate and compliant outputs.
How could generative AI change finance?
Generative AI holds significant potential for the finance industry, but its enterprise deployment must be carefully approached.
With the proper governance, fine-tuned models can automate tasks like risk analysis, reporting, and document summarization, all within secure environments that protect sensitive data and meet compliance standards.
The key benefits of a generative AI approach are reduced manual workloads, faster insights, and more strategic, data-driven decisions.
Here are a few high-impact opportunities:
- Early risk detection through transaction pattern analysis and market behavior.
- Automated reporting outputs that include presentation-ready narratives and visuals.
- Real-time scenario modeling to simulate budget outcomes as data changes.
- Compliance scanning to flag potential misalignments in regulatory documents.
- Investor communication support, including draft commentary and tailored Q&A preparation.
Generative AI applications for finance
Generative AI can automate tasks, accelerate analysis, and improve decision-making. From forecasting and reporting through to fraud detection and investor relations, its use cases span every corner of the finance function, for greater speed, accuracy, and impact. Here are a few applications.
Credit reviews
Generative AI is enabling finance teams to conduct faster and more accurate credit risk assessments by analyzing large volumes of structured and unstructured data. With a private-deployable model, it is even possible to integrate securely with internal credit risk systems and reference regulatory texts for accurate, policy-based outputs.
This means the AI can evaluate customer behavior, transaction history, and external market signals in real-time, allowing institutions to make more educated lending decisions.
By simulating various credit scenarios and flagging irregularities early, AI helps ensure decisions are both consistent and compliant.
Applications include:
- Anomaly detection: Spotting early fraud signals.
- Real-time credit assessment: Evaluating behavior and market data to adjust credit limits dynamically.
- Contract analysis: Extracting credit terms and obligations from large documents.
- Scenario modeling: Simulating different customer or market scenarios to predict risk.
- Policy lookup: Pulling relevant regulations or internal frameworks to support audits.
Accounting and financial reporting
Generative AI simplifies accounting functions by automating the creation of key reporting materials, like earnings summaries, financial commentary, sustainability filings, or stakeholder updates.
Using long-context models, AI can maintain consistency with brand tone and regulatory language, reducing the load on analysts and controllers.
This means teams no longer need to compile reports manually; AI can transform raw data into structured, presentation-ready outputs that can be refined for publication.
Applications include:
- Automated reporting: Populating report templates with real-time financial data.
- Narrative generation: Writing first drafts of earnings calls or financial updates.
- Regulatory alignment: Flagging discrepancies against accounting standards.
- Revenue recognition: Extracting payment terms and applying accounting treatments.
- Journal automation: Handling routine reconciliations and entries at scale.
Finance planning and performance management
Many planning cycles still rely heavily on historical data. Instead, generative AI’s intelligent planning capabilities can dynamically build and execute modeling strategies, ideal for complex performance forecasting.
When AI integrates with CRM, ERP, or market feeds, it surfaces trends and inefficiencies that could have been previously buried in spreadsheets. The result is more agile planning cycles and more time spent on strategic initiatives.
Applications include:
- Scenario simulation: Generating ‘what-if’ forecasts based on real-time data.
- Forecasting and budgeting: Producing dynamic budgets and adjusting as needed.
- Performance analysis: Highlighting variances and offering potential corrective actions.
- Visual reporting: Embedding charts, insights, and narrative into planning documents.
- Long-range modeling: Testing assumptions and refining strategy for high confidence.
Investor relations
Finance teams can also communicate more effectively with shareholders thanks to generative AI.
Whether drafting earnings scripts or preparing for potential inquiries, generative AI supports fast, consistent, and compliant messaging. This could range from support for investor Q&A generation to a sentiment analysis, ideally to proactively prepare for earnings calls and other shareholder engagements.
It’s also important to note that with RAG, AI can blend internal financials with market intelligence to tailor communication for specific investor audiences, while helping teams stay aligned with disclosure requirements.
Applications include:
- Earnings call preparation: Drafting scripts, commentary, and responses to common questions.
- Market insight generation: Surfacing competitor or sentiment trends to inform messaging.
- Activist simulation: Identifying concerns early using public and internal data.
- Visual asset creation: Designing investor decks and data visuals at speed.
- Regulatory research: Instantly retrieving policy details to support disclosures.
Generating business intelligence and risk mitigation
Generative AI extends what’s possible in business intelligence by surfacing insights from massive, often disconnected datasets.
RAG-enhanced systems enable near-instantaneous analysis across enterprise knowledge stores, helping finance teams respond to live market shifts, all without waiting weeks for detailed analysis.
Finance leaders can query data directly, generate custom reports, and visualize findings without needing coding skills.
Applications include:
- Trend analysis: Monitoring key metrics and identifying deviations in real time
- Risk detection: Spotting anomalies in spend, behavior, or market signals.
- Custom dashboards: Producing on-demand reports and visuals via natural-language input.
- Competitive intelligence: Extracting relevant insights from external data sources.
- Automated commentary: Generating summaries that explain changes and suggest action.
Real-world examples of generative AI in finance
Financial institutions are already seeing meaningful returns from generative AI.
At JPMorgan Chase, generative AI is being embedded across global operations. Its proprietary LLM Suite supports 50,000 employees with ideation, summarization, and research and is used in fraud prevention, investment analysis, marketing, and risk management.
Oracle Financial Services is using generative AI to modernize financial crime investigations. Its AI-powered Investigation Hub uses agentic workflows to guide analysts through complex investigations, automatically highlighting critical evidence and generating detailed summaries of suspicious activity.
Benefits of generative AI in finance
Generative AI can imitate expert reasoning, synthesize large datasets, and produce on-brand, context-rich financial outputs in real time. In particular, low-latency, long-context AI that’s deployed under strict governance can be a powerful ally for finance teams. Here are a few core benefits:
Operational efficiency
By automating time-intensive tasks such as forecasting, reporting, and reconciliation, generative AI enables finance teams to accelerate key workflows. Instead of manually compiling data and formatting reports, analysts can focus on strategic thinking and value-adding work.
Faster access to insights
Finance leaders no longer need to wait days for performance dashboards or risk reports, natural language queries can return insights in seconds. This speed empowers faster decisions and creates a more agile finance function overall.
Real-time personalization
Because generative AI adapts its outputs in response to new inputs, forecasts and recommendations can be updated dynamically as market data changes. For customer-facing teams, AI can tailor financial product suggestions based on individual behavior and preferences, strengthening engagement.
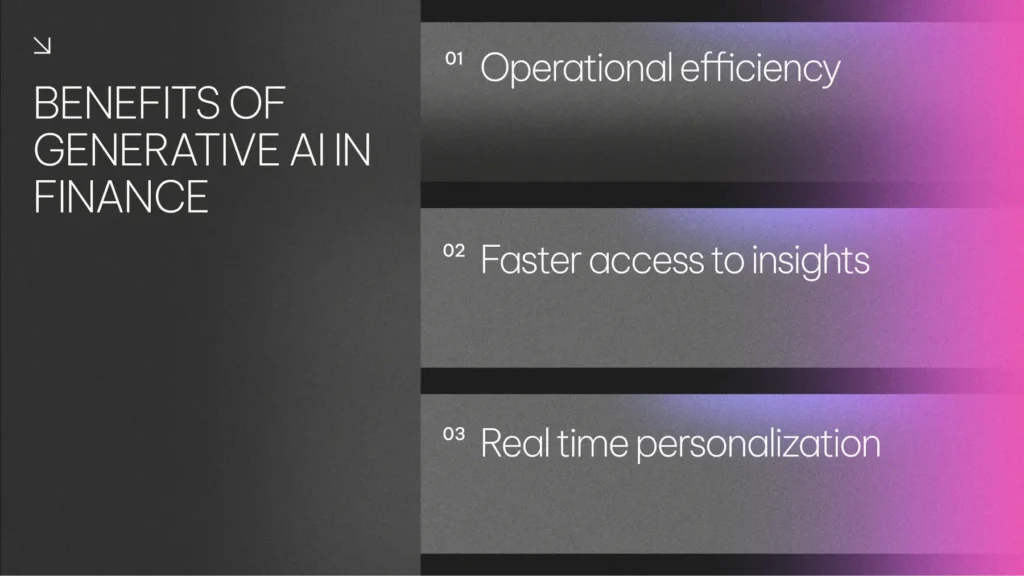
Limitations of generative AI in finance
Generative AI is powerful, but its outputs are not always accurate, and it’s essential for teams to apply strong oversight and maintain clear boundaries around its use.
Data privacy
Handling sensitive customer and financial information means privacy is non-negotiable. Without strict controls, generative AI could unintentionally expose confidential data during training or inference. That’s why finance businesses must take a ‘privacy-by-design’ approach and ensure that models are deployed securely.
Model hallucinations and data bias
Generative AI predicts based on learned patterns, not logic. It can generate plausible but incorrect information, particularly if the training data is biased or outdated. This can lead to errors in reporting, risk assessments, or strategic recommendations. To overcome this, human validation must be utilized at every step.
Opaque decision-making
Unlike traditional rule-based systems, generative AI models function more like black boxes. They produce outputs that may be difficult to trace or explain, posing a challenge in audit-intensive finance environments. A lack of explainability can undermine confidence and make compliance difficult, especially when financial models require transparency and defensibility. Again, human oversight is of critical importance.
Getting started with generative AI in finance
It’s generally recommended to launch privately deployed, task-specific models, which can run inside secure VPCs and align closely with internal systems, ideal for regulated finance environments.
It’s critical to define each model’s task clearly before deployment. (Models trained to perform a single function are easier to govern and faster to deploy, and they tend to be easier to align with internal systems. Many can even be deployed with on-premise infrastructure, ensuring compliance with strict data security standards.)
What is the future of AI in financial services?
The ultimate vision of enterprise AI is one that combines research-level innovation with real-world performance, freeing up human team members. In finance, these more accurate insight-led solutions will make it easier for finance teams to anticipate risks, seize opportunities, and communicate effectively.
Ultimately, the goal isn’t to replace finance professionals, but to empower them. However, this requires clean, centralized data; strong governance frameworks; and a clear understanding of how and where AI should be applied. Without quality inputs and oversight, even the most powerful AI system can produce misleading or risky outputs.
The takeaway for today? For finance leaders, the path forward with generative AI begins with clear goals, firm governance, and opting for the best task-specific models.