Table of Contents
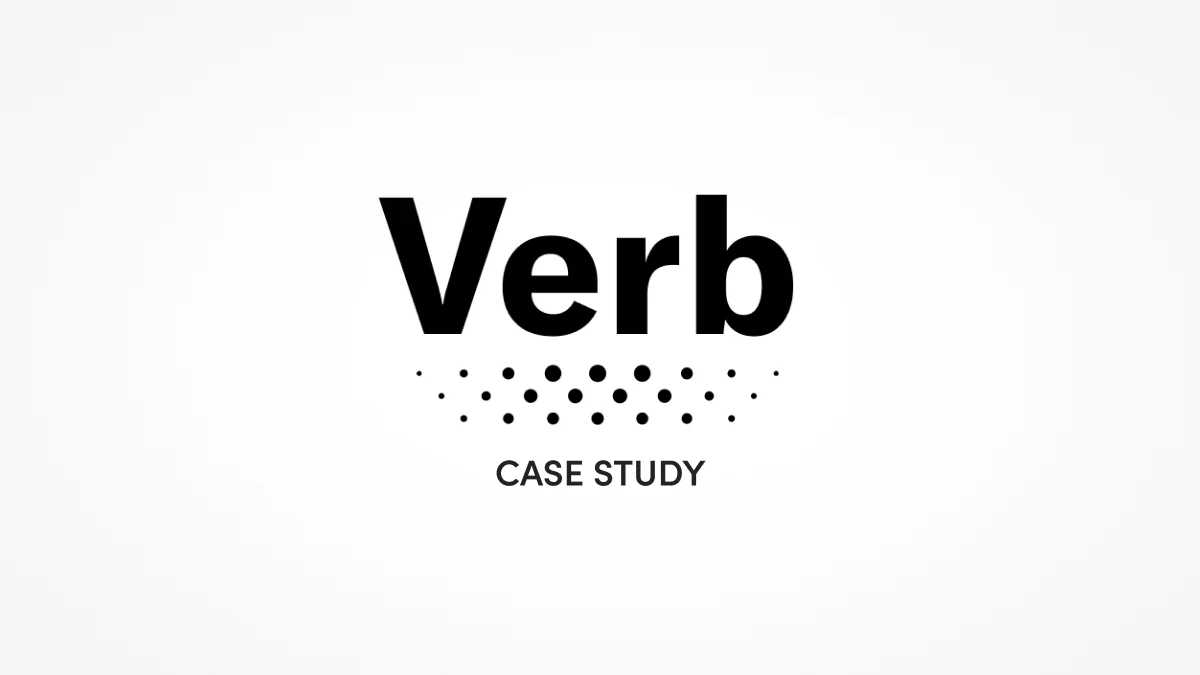
How Verb created a game-changing author tool with AI21 Studio
According to a New York Times article, 81 percent of Americans feel they have a book in them that they should write. Now, it may be an old statistic, but mankind’s desire to share stories has been around since the dawn of time as a way to share empathy, connection, and entertainment. So, it surely isn’t leaving human nature anytime soon.
But at the risk of stating the obvious: writing a book is hard. In fact, the writer of the aforementioned article, an author of 14 books, says “without attempting to overdo the drama of the difficulty of writing … composing a book is almost always to feel oneself in a state of confusion, doubt and mental imprisonment.”
Yeah, it’s a lot of hard work.
So how did AI21 Studio help Verb, an AI-enhanced tool for fiction writers, create a cutting-edge application designed to help writers and hobbyists alike complete their novels without all the hassle and uncertainty?
We spoke to Ryan Bowman, publishing and creative writing veteran and part of Verb’s small team of founders, to find out how AI21 Studio was a key to such creative writing success.
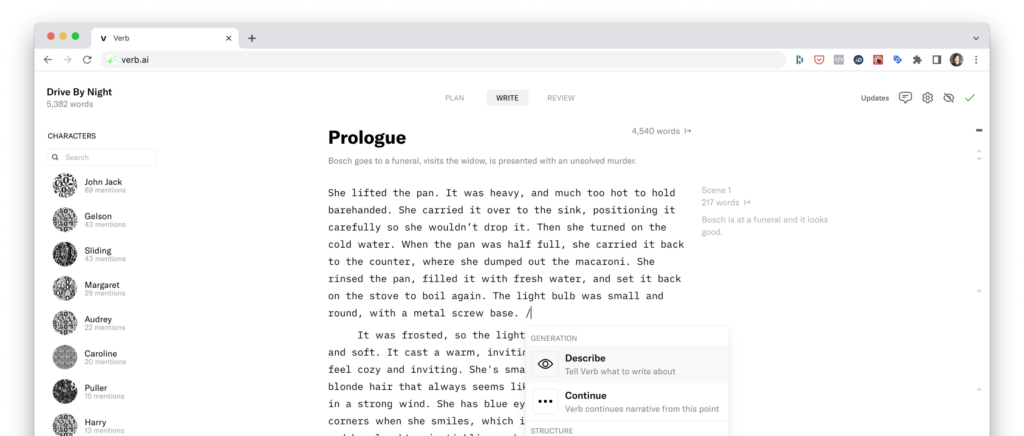
Forging the Way toward a New Creative AI Tool
Verb is a writing tool that helps make completing long-form narratives – novels for now but later films, TV shows, even video games – faster, easier, and more fun. It does so by assisting with all key stages of creation: brainstorming, writing, and editing. But all that wasn’t plausible until they found the perfect Language Learning Model (LLM). Let’s dive in further.
Use Case #1: Brainstorming Plot Points
As we saw in the New York Times quote above, keeping an entire story straight inside your head can be a lot of work. As anyone who’s tried to write long-form anything knows, it can get messy, convoluted, jumbled, and sometimes, uninspiring.
“They say novel writing is a group activity done by yourself,” Ryan tells us. “So one of Verb’s jobs is to be that other person, that brainstorm partner.” That’s why they set out to create one of their most important – and as it soon turned out, most popular – functions inside of their app: brainstorming. This feature allows you to plan the novel scene by scene, chapter by chapter.
That type of brainstorming could look like the following:
- You write: “Ben is sitting in his office and a woman appears next door.”
- You click: “Suggest a plot point”
- AI generates: “The woman pulls out a gun and chases him through the hallway.”
Then, you can either:
- Run with that idea in your scene and use it as the next plot point, or
- Continue clicking the “What happens next” button to generate more ideas until you find one that sparks your imagination and helps you continue.
An incredibly useful tool for writers, it’s been something that keeps these authors coming back for more. “One of the most amazing findings we’ve seen,” adds Ryan, “is that the more you use what makes Verb special – AI features like plot planning, the more likely you are to come back and write.”
Use Case #2: Writing Literary Language
Generative AI models are incredibly diverse, and capable of outputting new content, summaries, translations, answers to questions, and more. But one thing they haven’t been able to do just yet is write literary works. That’s to say, these AI models can create – but they’re not necessarily creative.
“We needed to build tools that are specific to the problems of a novelist. It couldn’t be just about generating text,” Ryan explains, noting that Verb knew the AI model they were to use must be able to generate discursive literary language.
“Language Learning Models (LLM) are not natural storytellers,” says Ryan, going on to explain how much storytelling is a learned craft; something that must be practiced for years in order to call it a skill. “There is 2,000 years of literary and practical theory backing up the idea that telling stories is hard – important and necessary but difficult.”
Luckily, Ryan and the team at Verb found the literary solution they needed in AI21 Studio. “When generating texts for our users who are looking for more discursive, more literary, more discriminating language, AI21 turned out to have a good tone and feel,” he says.
Use Case #3: Fine-Tuning, Iterations & Customer Support
One of the critical tasks of validating generative models is having criteria. To validate the reliability of this sort of generative AI effectively, you still need human involvement – especially when creating something brand new to the market.
How’d Verb achieve this? Verb built a platform that tests generative output amongst thousands of judgments across multiple writing professionals. These individuals look at different paragraphs of literary texts and decide which ones they like better, which ultimately helps Verb build a gradient of quality.
“We keep trying and testing and building iterations on top,” says Ryan, noting that they are always adding options to see which ones humans prefer. “As crazy as it sounds, we are in the process of teaching it literary theory.”
Along the same lines, it’s important to note that creating something so new to the market doesn’t just call for multiple iterations but also gathers support from everyone involved.
“We’re in a world of newness,” Ryan laments. “There is nothing like [Verb] so we’re not always sure what we’re supposed to be doing – it is freeing and slightly frightening at the same time.”
Ryan and the team at Verb knew they needed a company that could see in their vision – which is when they turned to AI21 Studio. “We found working with AI21 to be a breath of fresh air,” says Ryan, noting that it felt like a collaboration done with real human faces they can interact with for guidance, assistance, and ideas – rather than a business-client relationship.
The Results: More Authors Finishing their Novels, Faster
The self-publishing landscape of 2022 shows that indie writers are becoming the new norm. But AI has been an alleged threat to writers since its inception, leading many people to believe that writers wouldn’t even want the help of an AI-enhanced tool to get them across the finish line.
So, of course, Verb also has to ask themselves the question: Are writers really interested in having a machine collaborator help them with their creative writing? “Our early data says that they are,” says Ryan, noting that since launching its alpha version using AI21 Studio, Verb has seen a dramatic uptake of this experimental tool.
Not to mention, as we saw with the brainstorming feature, the more creative and collaborative the model, the more likely writers are to stick around the app.
In fact, Ryan confirms that there are a dozen or so authors (out of an intimate group of alpha users) who have done the hardest task – actually finishing their book! – stating that Verb helped them do just that.
With Verb, and the help of AI21 Studio, it can be done. Dare we say – it can be done faster, easier, and with more fun.
Verb.ai & Creative Texts in the Future
Verb launched its beta version in late December 2022 to a select number of users, and plans to use this custom model to expand into other narrative forms such as screenwriting and TV & Film.
With the pairing of new tools such as Verb with custom models from AI21 Studio, generative AI has evolved from just helping individuals generate text to something much more intertwined with the human love of storytelling; an AI tool that is not a threat to writers but actually a collaborator, a copy editor, a creative director, and even a confidante for inspired wordsmiths around the world.
Are you interested in building your own custom model with AI21 Studio by your side? Sign up for a free account here.