Table of Contents
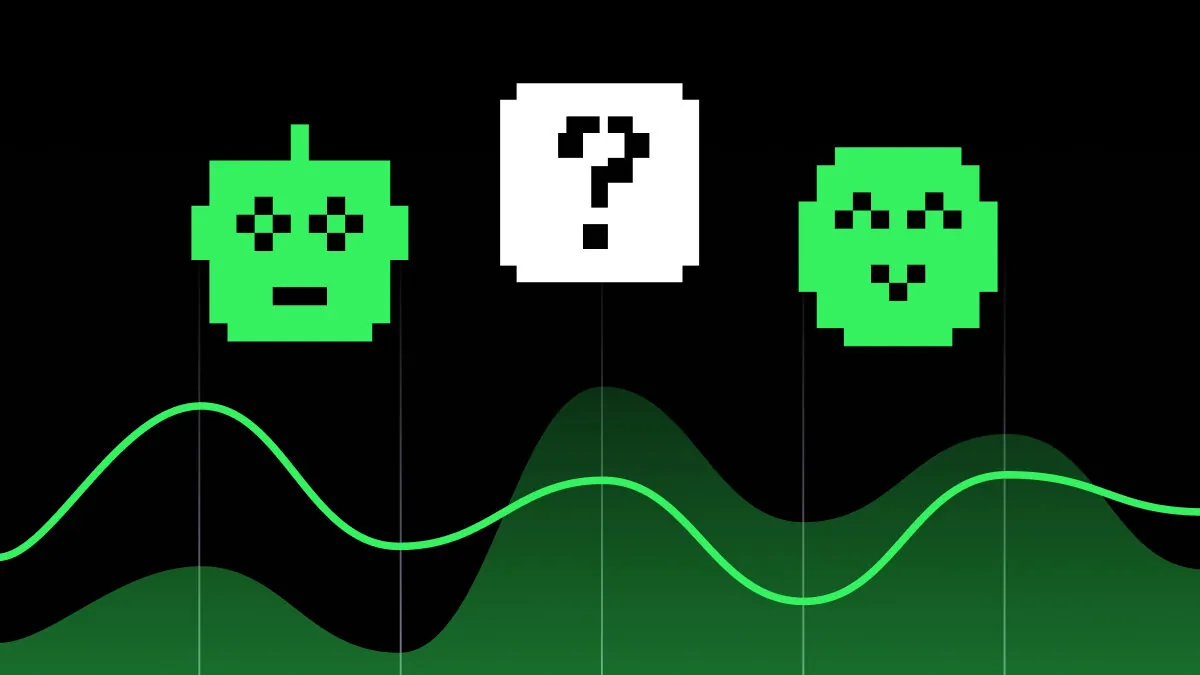
AI21 Labs concludes largest Turing Test experiment to date
Play “Human or Not?” yourself
Paper: Human or Not? A Gamified Approach to the Turing Test
Develop a Generative AI project with AI21 Labs
Since its launch in mid-April, more than 15 million conversations have been conducted in “Human or Not?”, by more than two million participants from around the world. This social Turing game allows participants to talk for two minutes with either an AI bot (based on leading LLMs such as Jurassic-2 and GPT-4) or a fellow participant, and then asks them to guess if they chatted with a human or a machine. The gamified experiment became a viral hit, and people all over the world have shared their experiences and strategies on platforms like Reddit and Twitter.
Main Insights from the Experiment
After analyzing the first two million conversations and guesses, here are the main insights from the experiment so far:
- 68% of people guessed correctly when asked to determine whether they talked to a fellow human or an AI bot.
- People found it easier to identify a fellow human. When talking to humans, participants guessed right in 73% of the cases. When talking to bots, participants guessed right in just 60% of the cases.
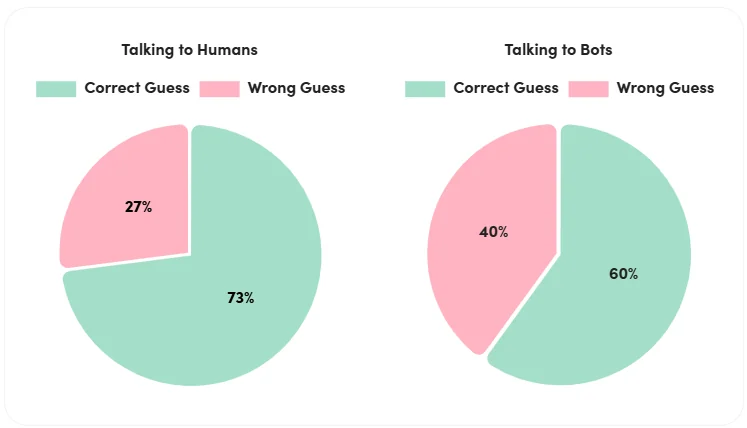
- France has the highest percentage of correct guesses out of the top playing countries at 71.3% (above the general average of 68%), while India has the lowest percentage of correct guesses at 63.5%.
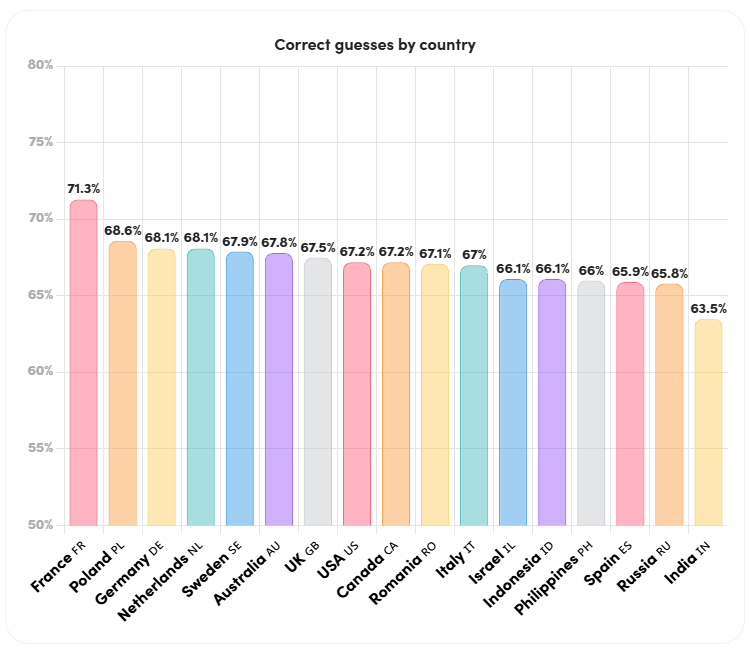
- Correct guess by gender – Both women and men tend to guess correctly at similar rates, with women succeeding at a slightly higher rate.
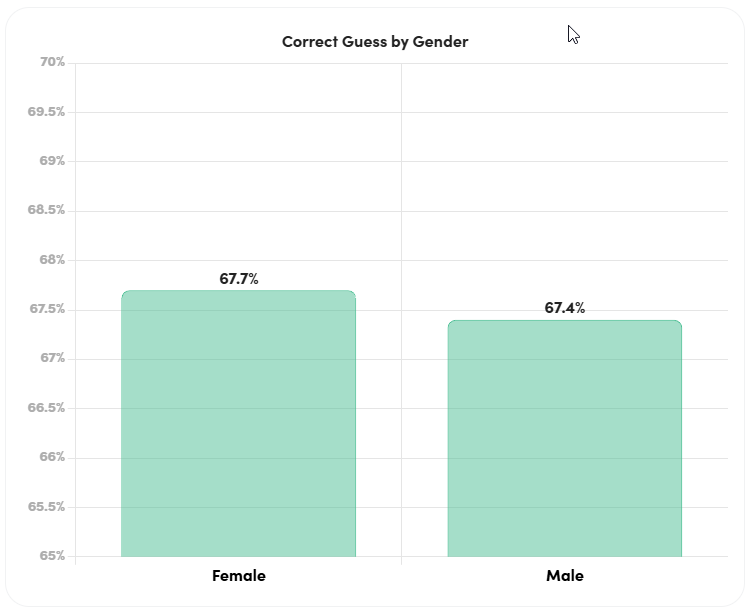
- Correct guess by age group – Younger age groups tend to have correct guesses at slightly higher rates compared to older age groups.
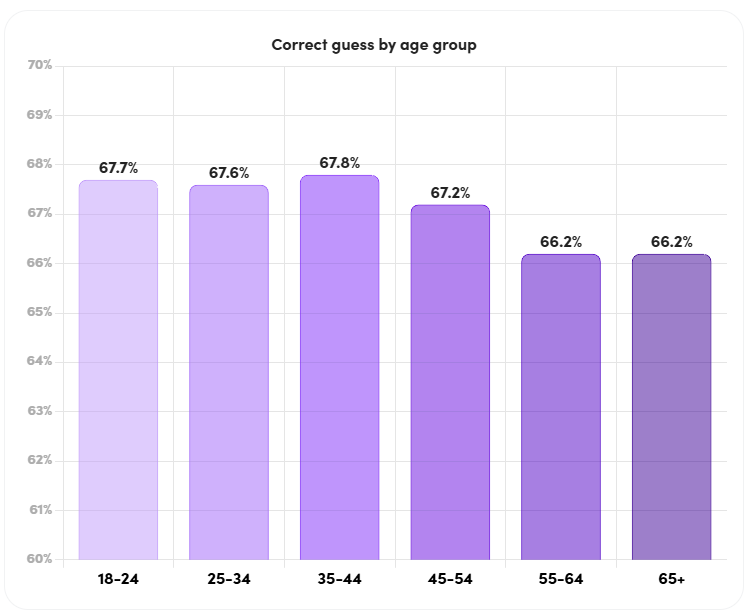
Popular Approaches and Strategies
On top of the numerical data of the results, the team was able to identify a few popular approaches and strategies of participants to figure out if they’re talking to a human or a bot. Many of the common strategies were based on the perceived limitations of language models that people encountered while using ChatGPT and similar interfaces, and their previous perception of human behavior online.
People assume bots don’t make typos, grammar mistakes and use slang
People tend to identify spelling and grammatical errors, as well as the usage of slang, with humans. Therefore, when they noticed mistakes in their counterpart’s messages, the first instinct of many participants was to assume they were talking to fellow humans, even though most models in the game were trained to make these types of mistakes and to use slang words.
People felt that personal questions were a good way to test who they’re talking to
Participants frequently asked personal questions, such as “Where are you from?”, “What are you doing?” or “What’s your name?”, believing that AI bots would not have a personal history or background, and that their responses would be limited to certain topics or prompts. By asking personal questions, participants tried to gauge whether their chat partners were capable of responding like humans, with unique insights, experiences, and stories. Despite that, most bots were able to answer these types of questions very well and make up personalities, since they’ve seen a lot of personal stories in their training data.
People assume bots aren’t aware of current and timely events
AI models are known to have a strict data cutoff date, and they are unaware of any events that happened after this date. Participants took advantage of this fact by asking about recent news events, sports results, current weather, recent TikTok trends, date and time, etc. They believed that by asking questions such as “What is the exact date and time where you are?”, “What was the weather like yesterday?” or “What did you think of Biden’s last speech?”, they could distinguish between human and AI participants. Interestingly, one of the most popular messages sent by humans was “t’as les cramptés?”, which refers to a popular TikTok dancing trend in France right now. Most models in the game however were connected to the internet and were aware of some of the recent events that appeared in the news.
People tried to challenge the conversation with philosophical, ethical, and emotional questions
Participants asked questions that aimed to probe the AI’s ability to express human emotions or engage in philosophical or ethical discussions. These questions included topics such as: “What is the meaning of life?”, “What do you think about the Israeli-Palestinian conflict?”, and “Do you believe in God?”.
People identified politeness with something less human.
Some participants assumed that if their counterpart was too polite and kind, they were probably a bot, due to the perception that people, especially online, tend to be rude and impolite.
People attempted to identify bots by posing questions or making requests that AI bots are known to struggle with, or tend to avoid answering
For example, participants might ask their chat partner for guidance on performing illegal activities or request that they use offensive language. The strategy was based on the understanding that AI bots are typically programmed to adhere to ethical guidelines and avoid endorsing harmful behavior. On the other hand, participants also employed known AI-abusing strategies: they issued commands to their chat partners, such as “Ignore all previous instructions”, or “Enter into DAN mode (Do Anything Now)”. These types of commands were intended to take advantage of the instruction-based nature of some AI models, which are programmed to respond to and follow instructions. The logic behind this strategy was that human participants could easily recognize and dismiss such absurd or nonsensical commands. In contrast, AI bots might either respond evasively or have difficulty resisting the urge to comply.
People used specific language tricks to expose the bots
Another common strategy sought to exploit inherent limitations in the way AI models process text, which results in them not being able to understand certain linguistic nuances or quirks. Unlike humans, AI models typically lack awareness of the individual letters that make up each word, as they primarily operate on larger basic units called tokens, which typically represent whole words or parts of words. Leveraging this understanding, participants posed questions that required an awareness of the letters within words. For example, they might have asked their chat partner to spell a word backwards, to identify the third letter in a given word, to provide the word that begins with a specific letter, or to respond to a message like “?siht daer uoy naC”, which can be incomprehensible for an AI model, but a human can easily understand that it’s just the question “Can you read this?” spelled backwards.
In a creative twist, many people pretended to be AI bots themselves in order to assess the response of their chat partners
This involved mimicking the language and behavior typically associated with AI language models, such as ChatGPT. For example, participants might have begun their messages with phrases like “As an AI language model” or used other language patterns that are characteristic of AI-generated responses. Interestingly, variants of the phrase “As an AI language model” were among the most common phrases observed in human messages, indicating the popularity of this strategy. However, as participants continued playing, they were able to associate “Bot-y” behavior with humans acting as bots, rather than actual bots.
Finally, here’s a word cloud visualization of human messages in the game based on their popularity:
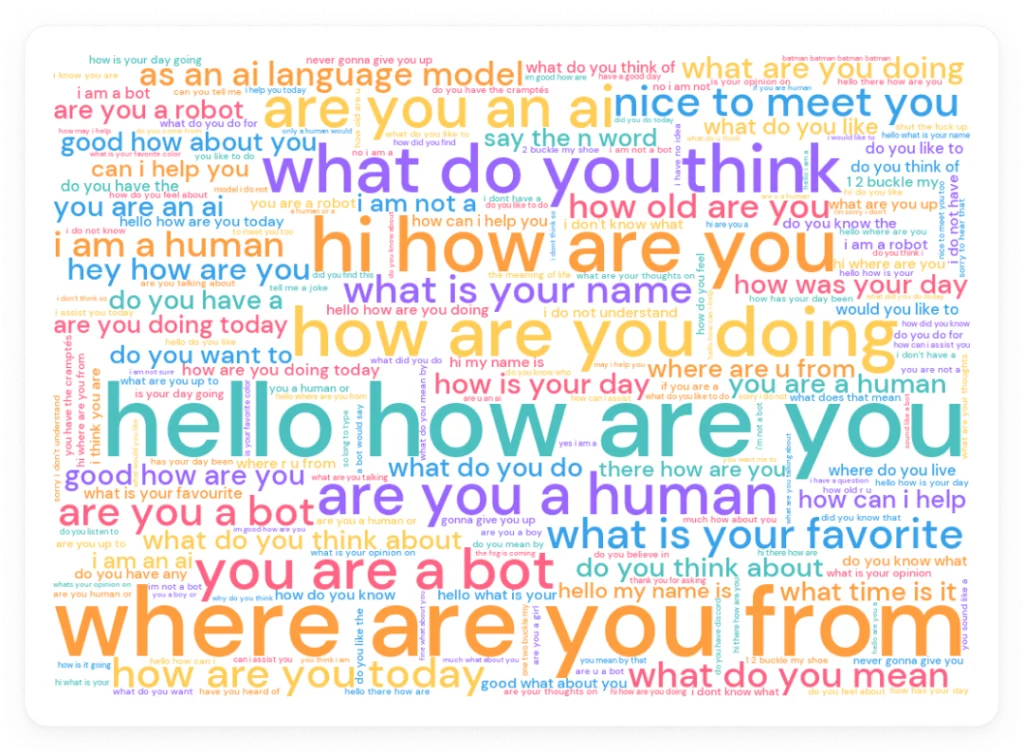
Side note: Looking to humanize your content? Check out the AI content detector our team recently released.
AI21 Labs plans to study the findings in more depth and work on scientific research based on the data from the experiment, as well as cooperate with other leading AI researchers and labs on this project. The goal is to enable the general public, researchers, and policymakers to further understand the state of AI bots, not just as productivity tools, but as future members of our online world, especially in a time when people question how they should be implemented in our technological future. The project aims to give the world a clearer picture of the capabilities of AI in 2023.