Table of Contents
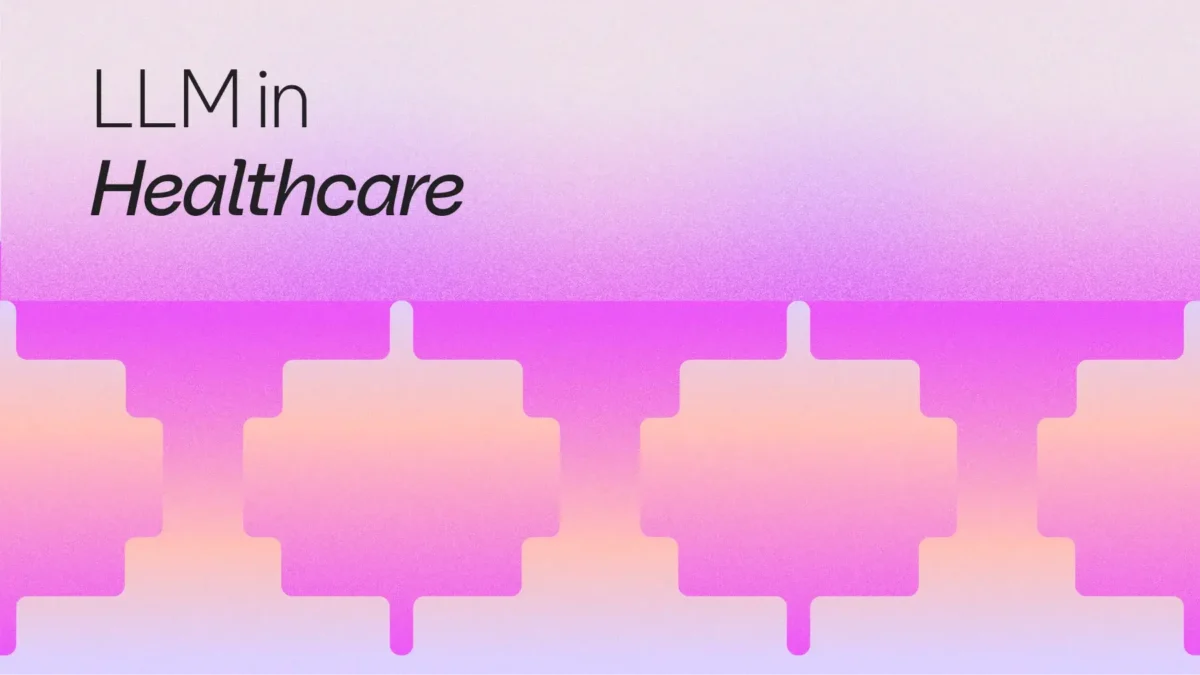
LLMs in Healthcare: Applications, Examples, & Benefits
Patients now wait an average of 26 days to see a new doctor, the longest wait time ever recorded. While the demand for timely and personalized healthcare is rising, clinical burnout, staffing shortages, and administrative overload are compounding the issue, widening access gaps, and compromising patient outcomes.
At the same time, vast volumes of unstructured clinical data go underutilized, buried in fragmented systems that slow diagnosis and strain operations. Without the right tools, even well-staffed healthcare organizations can miss early indicators, delay care decisions, and risk preventable outcomes.
Large language models (LLMs) offer a new approach, enabling the synthesis of clinical notes, surfacing insights from research, and streamlining documentation in seconds.
But adoption in healthcare isn’t just about what’s possible. It’s about what’s responsible. In this article, we explain how to deploy them safely and effectively.
What is an LLM in healthcare?
A large language model (LLM) is an advanced type of artificial intelligence trained to understand and generate human language. LLMs in healthcare can be trained on clinical notes, research papers, and patient records, from which they can summarize documents, analyze medical data, extract key information, and support clinical decision-making.
Long-context models, in particular, are able to analyze extensive, unstructured clinical text in a single pass, thereby reducing fragmentation in documentation tasks.
By processing this complex, unstructured text, healthcare professionals can access relevant insights more efficiently, improving diagnostic accuracy and enhancing patient care.
How can LLMs support the healthcare industry?
LLMs not only support patient and healthcare provider relationships but can also enhance access to scientific knowledge, facilitate ongoing medical learning, assist in drug discovery, and inform treatment planning.
- LLMs interpret medical images, predicting outcomes such as hospital readmissions, as well as advanced fields like genomics and personalized medicine.
- In patient care, LLMs offer personalized treatment recommendations, as well as a way to translate complex medical information, and power virtual health assistants that provide continuous, responsive support. This accessibility can also improve clinical trial enrollment processes.
- Open-source LLMs that offer cutting-edge, long-context AI are built for accuracy, efficiency, and powerful text generation, making them ideal for healthcare organizations seeking greater customization and control.
Key roles for an LLM in healthcare
A review of eighty-nine studies across twenty-nine specialties found that LLMs are already supporting patient care by answering patient questions, summarizing medical texts, translating complex information, and streamlining clinical documentation. Here are some other key roles for an LLM.
Enhancing diagnostic accuracy
LLMs support diagnostic decision-making by analyzing patient symptoms, medical records, and research data to provide evidence-based insights. Applications include radiology, oncology, and dermatology, identifying abnormalities and enhancing diagnostic interpretations.
Emerging multi-modal LLMs (models that combine visual and textual data) also show particular promise in enhancing medical imaging analysis.
Personalizing treatment plans
LLMs assist healthcare providers in developing more personalized treatment strategies. By analyzing patient symptoms, medical histories, and clinical data, and synthesizing large volumes of medical literature and case records, LLMs provide evidence-based insights for tailored interventions tailored to individual needs.
When trained, LLMs answer complex medical questions, supporting healthcare teams in selecting more targeted therapies that might be typically overlooked.
Optimizing operational efficiencies
Administrative tasks can be alleviated by large language models. Examples include capturing and summarizing physician-patient conversations, enhancing insurance coding and billing support, and generating required clinical notes. Even simple tasks, such as drafting prescriptions, producing referral letters, and creating standardized discharge summaries, enable healthcare professionals to focus more time on patient care.
LLM applications for healthcare
Large language models have many valuable applications across healthcare systems, from diagnostic support to administrative automation. The next decision for healthcare leaders to make is what areas will provide the best benefits.
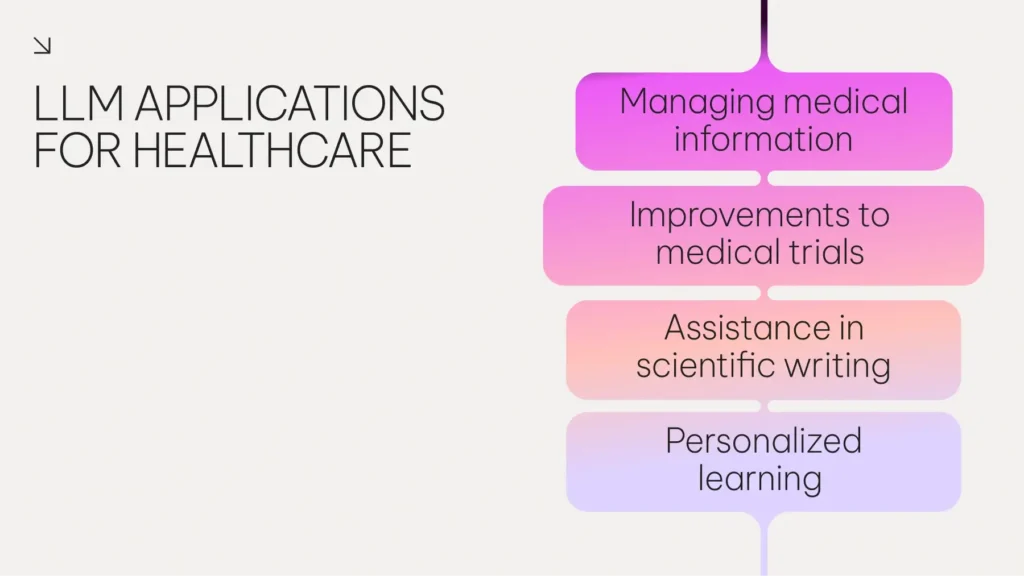
Managing medical information
LLMs analyze unstructured clinical notes to ensure that insights that might otherwise be missed are surfaced and tailored. Premium models that utilize grounded retrieval (responding based on the information contained in their knowledge base) can even analyze physician-free text notes.
A hospital system could use an LLM to automatically extract signs of early disease from physician notes, flagging at-risk patients sooner, and initiating preventive care.
In another example, a community health clinic could use an LLM-based system to generate insurance coding summaries from medical information immediately after patient visits, improving claim turnaround times.
Improvements to medical trials
Advances in large language models (LLMs) with expanded context windows are making communication with patients far simpler.
While healthcare chatbots are a good example, the communication improvements can also be found in areas such as the summarization of clinical trial interviews. With an LLM, a research team could summarize transcripts from multiple participant interviews, all at once, to accelerate publication timelines.
By processing large volumes of qualitative research data simultaneously, LLMs provide an opportunity to enhance evidence gathering, refine cohort analysis, and facilitate faster innovation in clinical trials.
Streamlined documentation
Healthcare organizations can use LLMs to streamline clinical documentation by automating note-taking, chart reviews, and summaries. One emerging application is prompt-triggered chart review and automated dictation.
For example, a healthcare provider can implement an LLM that listens to clinician voice prompts during patient exams and generates nearly complete visit notes, thereby reducing after-hours documentation time.
This could also be used to offer real-time data extraction and automated creation of discharge outlines, or to instantly convert post-surgical reports into structured summaries.
Assistance in scientific writing
LLMs have the potential to assist researchers in summarizing large volumes of scientific literature and can condense hundreds of research papers into key findings. LLMs also support researchers in streamlining scientific communication by generating clear reports.
A medical research team can use an LLM to draft based on clinical trial data, allowing them to complete submissions more quickly and efficiently. They could also use an LLM to translate complex research into more accessible formats for patient advocacy groups.
Personalized learning
LLMs are also powering personalized educational experiences for healthcare professionals and students. With an LLM, a teaching hospital can quickly create customized revision aids for medical residents, tailoring practice tests for exams to individual learning needs.
In more advanced cases, interactive learning simulations can also be used, with an LLM serving as a ‘virtual patient simulator’ to enable students to practice clinical interviews, diagnostic reasoning, and treatment planning in a low-risk environment.
Real-world examples of an LLM in healthcare
Large language models (LLMs) provide healthcare organizations with powerful tools to enhance operational efficiency, strengthen clinical decision support, and improve patient engagement, which are already being implemented in the industry.
AMIE
AMIE (Articulate Medical Intelligence Explore) is an AI system built on a large language model, designed for diagnostic medical conversations. It can take clinical histories, ask follow-up questions, explain reasoning, and respond empathetically. In a controlled study, AMIE outperformed primary care physicians in terms of diagnostic accuracy and communication quality across 149 simulated cases in multiple specialties.
Microsoft Healthcare Agent Service
Open source applications are also offering promise. The Microsoft Healthcare Agent Service enables developers to build compliant, AI-powered healthcare copilots. Because this is accomplished using LLMs and healthcare-adapted orchestrators (AI systems designed to manage clinical workflows and connect to trusted data sources), it can be used for tasks as vast as symptom checking, scheduling, and documentation.
Amazon Bedrock
Amazon Bedrock is another example of a method of accelerating LLM adoption, and over 10,000 organizations now use Amazon Bedrock’s foundation models globally, particularly to enhance care delivery. For example, Fujita Health University has automated discharge summaries, reducing processing time by 90%, while Genomics England utilizes an LLM to identify gene-disease links more efficiently.
Best practice for deploying LLMs in healthcare
The safe integration of LLMs into healthcare requires thoughtful planning, clear governance, and strong safeguards. Below are four key considerations for deploying large language models (LLMs) responsibly.
Set guidelines
Healthcare organizations must first establish clear policies on the use of LLMs.Responsible model use is imperative, and this can be done by distinguishing between high-risk clinical tasks and low-risk administrative use cases. For example, an LLM may be restricted from making critical diagnostic or treatment decisions without direct human oversight. Defining specific use cases up front helps minimize safety risks and maintain clinical accountability.
Use diverse data sets
Training and evaluating large language models (LLMs) on diverse datasets is crucial for reducing bias and enhancing model reliability. Since LLMs learn from their training data, reliance on narrow or biased sources lead to inequitable outcomes. Incorporating representative datasets is crucial, as is utilizing a model that facilitates the fine-tuning of proprietary datasets.
Transparent development
A transparent development process is essential for building trust among clinicians, patients, and regulators. Open dialogue about an LLM’s capabilities, limitations, and areas of uncertainty enables more informed use and ongoing evaluation. This starts by understanding how large language models (LLMs) generate their outputs, and recognizing where errors may occur is crucial for safe adoption in healthcare settings. A model with low hallucination rates (<3% is the level for AI21’s models, for example) ensures high trustworthiness in diagnostic contexts, especially important in regulated environments.
Choose open vs. closed source
The decision between using open-source or closed-source LLMs carries important trade-offs. Open-source models enable healthcare organizations to run systems on private servers, keeping patient data in-house and offering greater customization options. However, they require more technical expertise to maintain.
Closed-source models offer easier integration and vendor support but involve transmitting data externally and limit customization and transparency.
A tool such as Jamba, which offers an open-weight model with commercial licensing and enterprise-grade support, may represent a middle path between control and vendor-backed scalability.
The future of healthcare with LLMs
LLMs provide healthcare organizations a powerful opportunity to expand access, personalize care, and alleviate operational strain.
Yet success requires thoughtful planning, rigorous governance, and ongoing evaluation. Model selection is crucial, with the search ideally focusing on those that can operate in VPC or on-premises environments to preserve data privacy, ensure HIPAA compliance, and reduce exposure to third-party risks.
What is clear is that healthcare leaders who adopt a proactive, safety-first approach to LLMs can position their organizations to lead in a new era of adaptive, patient-centered healthcare.
FAQs
-
Large language models (LLMs) in healthcare are AI systems trained on extensive medical and general language data to understand, generate, and interpret text for a wide range of clinical and administrative tasks.
-
In healthcare and medicine, LLM stands for Large Language Model. These are artificial intelligence (AI) systems trained on massive volumes of text data, including medical literature, clinical notes, and patient records, to understand and generate human-like language for tasks such as summarizing patient histories, supporting diagnosis, automating documentation, and extracting insights from unstructured clinical data./