Table of Contents
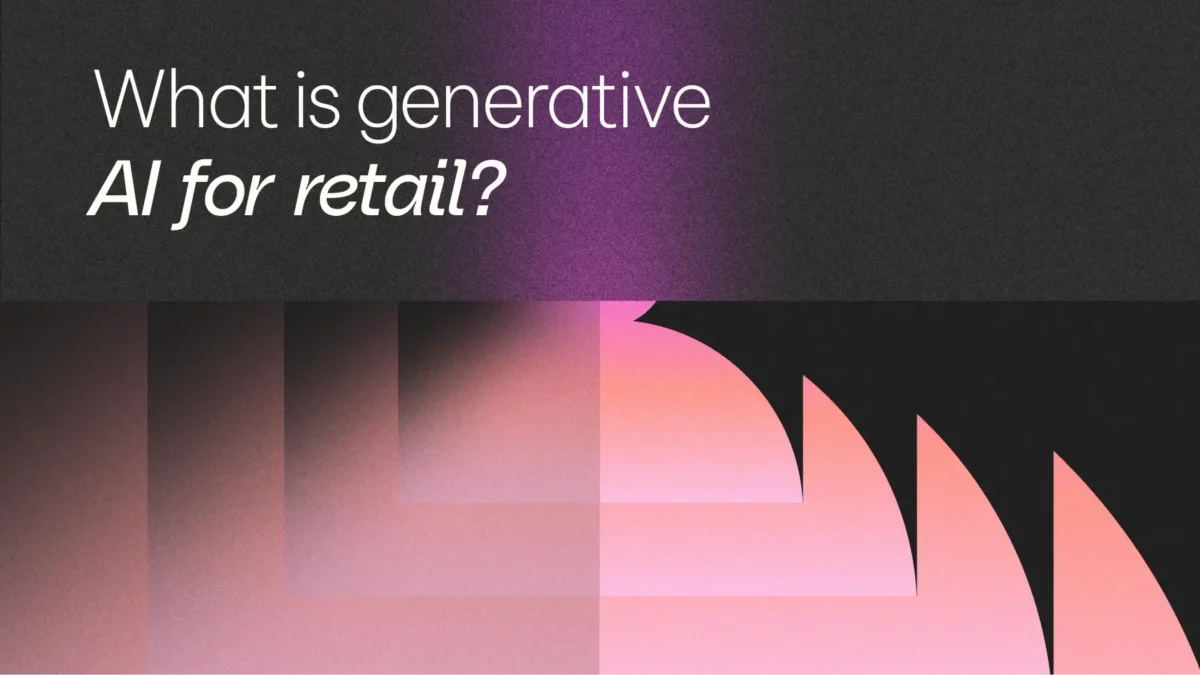
Generative AI in Retail: Applications, Examples & Benefits
Retailers are pressured to stay competitive in a fast-changing market, but many are falling short. While 89% are already using or piloting AI in some form, many are limited in genuine innovation due to infrastructure complexity, regulatory hurdles, or poor results from experimentation with general-purpose models.
However, when the right model is properly implemented, generative AI has the potential to boost revenue or streamline operations across the whole retail value chain.
A recent study found retailers saw a 69% revenue boost with AI adoption and a 72% decrease in operating costs. Another found that for every $1 an organization invests in generative AI, it’ll see an average of $3.70 in return.
Below, we’ll explore what’s possible for retailers with generative AI, including methods of adoption in marketing, commercialization, distribution, and back-office work.
What is generative AI for retail?
Generative AI refers to machine learning models that produce original content, text, images, video, or structured data based on learning patterns from vast training data or enterprise-specific datasets.
It even does this using unstructured data and can complete tasks such as:
- Generating product descriptions from supplier documents.
- Recommending products based on browsing behavior.
- Summarizing customer reviews for quick insights.
- Creating personalized marketing messages across channels.
Generative AI can also utilise Retrieval-Augmented Generation (RAG), a method that improves language models by pulling in real-time information from trusted sources. This means AI can generate more accurate and context-aware responses grounded in a retailer’s preferred sources.
How could generative AI change retail?
Among retailers with over $500 million in revenue, 64% have adopted AI, significantly higher than the industry-wide average.
This is just the start of the possibilities. Generative AI has the capabilities to help in areas such as:
- Improving in-store customer service by providing employees with quick access to product information.
- Automated content creation, including writing product descriptions or generating localized content.
- Synthesizing customer feedback from various sources to inform business decisions.
- Aiding product design by analyzing market trends.
Generative AI’s strength lies in its ability to automate tasks that traditionally required significant human effort, such as content creation and customer interaction, while simultaneously delivering personalized experiences at scale.
Generative AI applications for retail
Generative AI is transforming retail by streamlining operations, personalizing customer experiences, and unlocking new creative possibilities across the value chain.
Digital experiences
Generative AI powered by large language models (LLMs) can deliver accurate, on-brand responses in real time. By using Retrieval-Augmented Generation (RAG), these systems combine customer data with product knowledge to create more personalized and context-aware communication.
For example, if a customer asks, “What should I wear to a winter countryside wedding? I want to hide my arms,” a generative AI assistant can suggest suitable outfits and provide direct links to the shop.
Long-context models further enhance this experience by reducing hallucinations and grounding responses in verified data, which is essential for maintaining brand safety and regulatory compliance in customer-facing applications.
Applications include:
- Personalized recommendations: Suggesting products based on purchase history, browsing behavior, and preferences.
- Virtual stylists and avatars: Offering tailored outfit or product suggestions through AI-powered assistants.
- Conversational assistants: Answering customer queries with personalized, human-like responses.
Conversational commerce
Generative AI enables more natural and intuitive shopping interactions that feel like talking to a helpful in-store expert. Generative AI powers smart-search features that allow shoppers to ask natural language queries.
For example, a customer can use voice or text to state “I need a gift for a teenager who loves tech,” and receive curated product suggestions.
Often referred to as AI assistants or “copilots,” these systems utilize natural language processing (NLP) to comprehend context and provide meaningful, human-like responses.
They aren’t just for customers either; retail teams can also use generative copilots to query internal knowledge bases and receive instant summaries on product features, inventory levels, or from training guides.
Applications include:
- Shopping assistants: Recommending products via chat, voice, or image input.
- Gift finders: Understanding vague or complex requests and returning smart suggestions.
- Natural language search: Customers can ask plain English queries about products.
Automated content creation
Generative AI helps retail teams generate consistent content at scale and rapid speeds, reducing manual work while maintaining brand messaging consistency. For global retailers, this opens up faster localization campaigns, such as producing region-specific assets for websites, social media, PR, and off-site campaigns, all while maintaining brand consistency.
In particular, open models with structured JSON output (JavaScript Object Notation, a simple format used to structure and organize data so that computers can easily read and share it)
and the ability to undertake citation generation can help ensure the content that’s produced is accurate.
It can also handle functional content, such as shelf labels or SEO product descriptions, at scale. Beyond efficiency, generative AI allows brands to generate content in a consistent tone of voice or tailor it to different personas.
AI can even predict and produce the most compelling content based on customer preferences and purchase history, from subject lines to full email campaigns and personalized social media ads.
Applications include:
- Product descriptions: Writing SEO copy for thousands of SKUs.
- Localized content: Creating region-specific versions of campaigns or landing pages.
- Email and ad copy: Tailoring messaging to different customer segments or personas.
- Shelf labels and signage: Generating physical store content in bulk.
- Social media posts: Creating reactive, branded posts quickly.
Real-world examples of generative AI for retail
Retail leaders are already seeing measurable impact from generative AI.
Adidas reported it has streamlined its engineering team by 50% and shifted focus to in-house innovation, using generative AI’s ability to rapidly turn ideas into customer-facing features. It has reportedly enabled 4,000 digital releases per year, five times faster than before, while still growing online revenue by 10% year over year, contributing $5 billion across more than 80 countries.
Meanwhile, Amazon is embedding generative AI within personalized shopping prompts that enable shoppers to use natural language for tailored product suggestions, while advertisers can use the AI Creative Studio to generate high-quality visuals, videos, and audio ads from simple product inputs.
The benefits of generative AI for retail
Generative AI can simulate human-like capabilities, producing context-rich text and visuals based on logic and pattern recognition. This opens it up to many growth areas retailers have traditionally struggled with.
Decreased operational costs
Using generative AI to automate tasks can lead to time and cost savings in areas like marketing, support, and content creation. By bringing repetitive tasks in-house that would typically have been outsourced, employees can spend their time on impactful projects and re-allocate budget towards customer acquisition.
Hyper-personalization
A study of over 1000 consumers found that 81% of customers prefer a personalized experience. Generative AI can analyze vast amounts of customer data, including purchase history, preferences, and browsing behavior, to create highly personalized experiences.
Improved customer service
A study of over 4 million brand ratings from 3,500 brands found that consumers are 4.4 percent more likely to do future business with companies considered customer service leaders.
Generative AI powers intelligent tools that enhance customer interactions, which can create a true 24/7 service experience that guides and supports customers through their buying journey.
The limitations of generative AI for retail
Generative AI is powerful, but it’s crucial to separate generative AI from true human intelligence.
While powerful, its use in retail must be approached with clear boundaries.
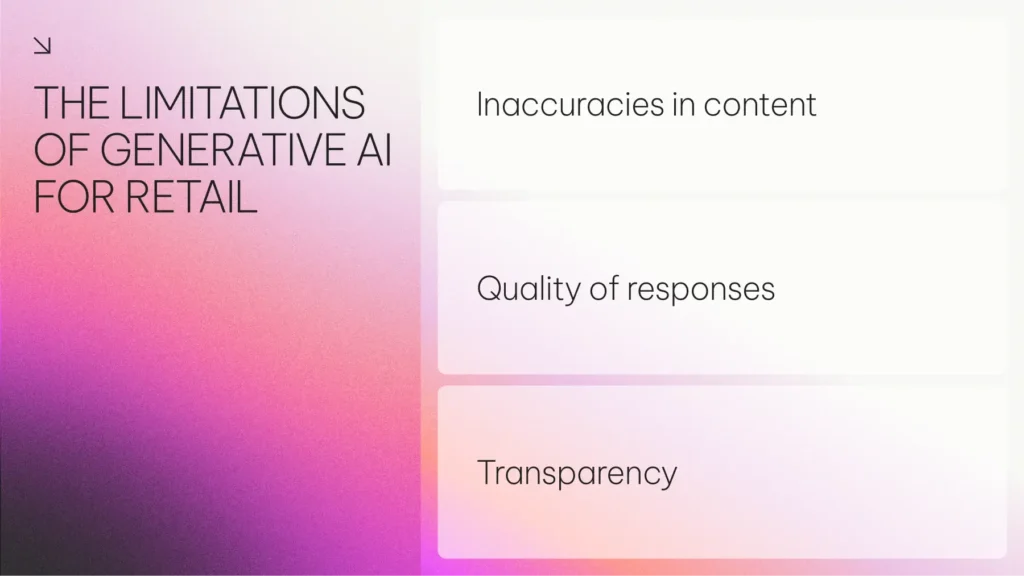
Inaccuracies in content
Generative models can produce convincing but incorrect information, commonly known as “hallucinations.”
In customer-facing scenarios, this could result in mislabelled product descriptions, inaccurate claims, or irrelevant content being shared online.
To reduce hallucinations and ensure the scale of application of AI, retailers must choose models that support long-context ingestion to enhance accuracy in data retrieval, even over long, multi-faceted queries, for example, a chat experience.
Quality of responses
Generated content, from trend insights to product copy, still needs human validation. Generative AI predicts the most likely response, but can default to more generic replies.
A way to reduce the risk is to use open-weight models, which can be privately fine-tuned on proprietary data, supporting secure and custom workflows like knowledge search, customer support, or RFP responses.
Transparency
Retailers must consider how to disclose the use of AI in content and communications, especially as consumers may increasingly demand clarity about what’s human and AI-generated.
Ethical AI usage policies are worth investigating to ensure that AI use is strategic and responsible in case questions crop up.
For retailers exploring generative AI, the first step is identifying where transformation will deliver the most value — whether in customer experience, marketing, or in-store productivity. But building and training a model from scratch can be costly, time-consuming, and technically demanding.
Task-specific models (TSMs) offer a practical solution. TSMs provide a faster, more focused alternative to general-purpose models. They are pre-trained models built for a single, defined task.
For example, a semantic search TSM allows shoppers to ask for products in their own words and receive results based on intent — not just exact phrasing.
A TSM designed for contextual answers responds to queries using a retailer’s trusted content, delivering accurate, brand-aligned responses.
Because TSMs are built around a single retailer’s source of truth, they’re easier to customize, require less training data, and avoid the complexity of general-purpose models.
Private AI deployment options also mean these AI assistants can run within secure virtual private clouds (VPCs) or on-premise environments — a critical consideration for retailers handling sensitive customer data or regulated transactions.
Will the future of retail be powered by gen AI?
The future of retail will become increasingly personalized, powered by generative AI models that are fine-tuned for specific retail tasks and able to work at speed.
Creative ideas will be delivered faster, and with ethical control and guardrails, retailers can use generative AI to support in areas as vast as tailored product search to AI-assisted product development.
However, the successful implementation of generative AI requires more than cutting-edge models. Retailers need both a strong foundation and strategy. That means ensuring data is clean, centralized, and governed.
Understanding outputs is key, and without robust, high-quality inputs, even the most advanced generative models can produce weak or inaccurate results, and it can be hard to backtrack.
Generative AI must work alongside existing tech stacks and customer journeys, ensuring the model fits with legacy systems. While the learning curve may sound steep, the retailers willing to invest in the right areas of generative AI will be shaping the next era of commerce.
FAQs
-
Generative AI is used in retail to create personalized content, automate customer support, enhance product discovery, and accelerate design and marketing processes.
-
AI is used in retail stores for real-time inventory tracking, dynamic pricing, staff scheduling, customer analytics, and interactive shopping experiences like virtual try-ons or smart displays./i